Description of the topics/core technologies
In the core technology cluster Anomaly Detection we are interested in methods that can detect anomalies in different kind of fields. Areas that are of special interest in our group are at the moment: Anomaly Detection, Machine Learning, Radar, Operations Data, Quality of Service, Generative Models, Deep Learning, Unsupervised/supervised and Semi supervised Learning, Edge Clouds, Sequential Modelling, Radio Access Networks, 5G.
Cluster activities
- Zoom meetings: one every second month
- Presentations of our work for each other
- Share of latest science concerning anomaly detection.
- Plan for a study trip
Contact all Members
Mailing List: CTC_AnomalyDetection@wasp-sweden.se
Cluster leader
Cluster members
Here is a list of our cluster members, read more about what we do and feel free to contact us.
- Affiliation: PhD Student at Linköping University, Department of Computer and Information Science (IDA)
- Main supervisor: Fredrik Lindsten
- Research Keywords: Out-of-Distribution Detection, Unsupervised and Self-Supervised Learning, Generative Models, Deep Learning
- Publications: https://scholar.google.com/citations?hl=en&user=CH5AZiIAAAAJ
- Email: amirhossein.ahmadian@liu.se
- Affiliation: KTH
- Main supervisor: Florian T. Pokorny
- Research keywords: networks, transactions, representation learning, topology, anomaly detection
- Research aim: To find statistical patterns of normal and abnormal behaviour in transaction networks
- Publications: https://scholar.google.com/citations?hl=en&user=pXzlVfkAAAAJ
- Email: ciwan@kth.se
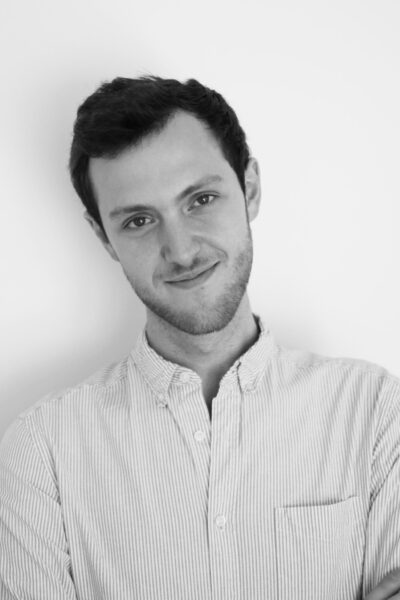
Ciwan Ceylan
- Affiliation: Umeå University (WASP academic Ph.D. student)
- Main supervisor: Erik Elmroth
- Research keywords: Anomaly Detection, Edge Clouds, Machine Learning, Deep Learning, Sequential Modelling, Data Analysis
- Research Abstract: “The focus of my research is on proposing and developing reliable anomaly detection systems for the edge clouds environment. With this aim, we mainly take advantage of learning-based solutions to address the security and performance anomalies that are specific to edge clouds and cause QoS degradation and resource outage.”
- Publications: https://scholar.google.com/citations?hl=en&user=sENcHGwAAAAJ
- Email: javadforough@gmail.com
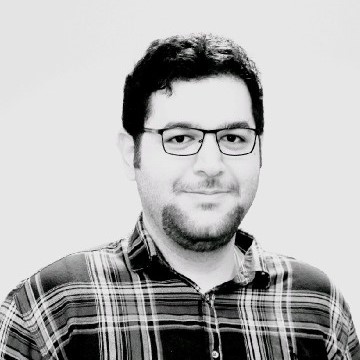
Javad Forough
- Affiliation: LTH, Department of Computer Science – WASP AS Batch 3
- Academic supervisors: Per Runeson, Emelie Engström
- Research keywords: development, operations, DevOps, operations data, design science, machine learning, anomaly detection
- Research aim: To increase engineering productivity and service quality by utilizing machine learning techniques for the analysis of operations data collected through the monitoring systems
- Publications:
- Email: adha.hrusto@cs.lth.se
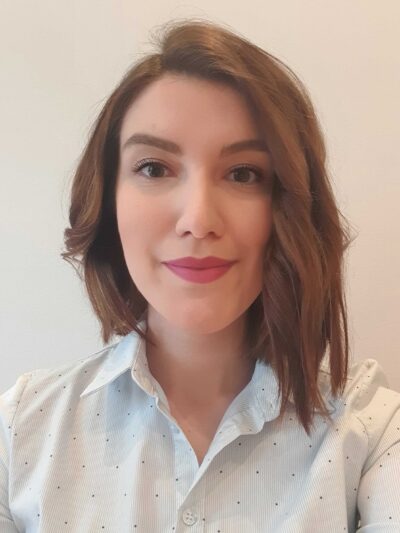
Adha Hrusto
- Affiliation: KTH
- Main supervisor: Magnus Jansson
- Research keywords: Radar and Machine Learning
- Research questions: Improving radar signal processing with neural networks and model based learning
- Publications:
- Email: alek@kth.se
- Affiliation: Industrial PhD at Umeå University and TietoEvry Umeå
- Main supervisor: Erik Elmroth
- Research keywords: Anomaly detection, Radio Access Network, root cause analtics, functional behvaiour, 5G, Observability.
- About my research: “The purpose of my research is to speed up the process of finding anomalies in the 5G Radio Access Network (RAN). Developers spends huge amount of time to manually analyze RAN system logs. By using different machine learning methods I automatically detect and find root causes of anomalies in the RAN system logs. In this way anomalies can be found earlier, faster and by less expertice.”
- Publications: https://scholar.google.com/citations?user=WciBrz4AAAAJ&hl=en&oi=ao
- Email:sundqtob@cs.umu.se
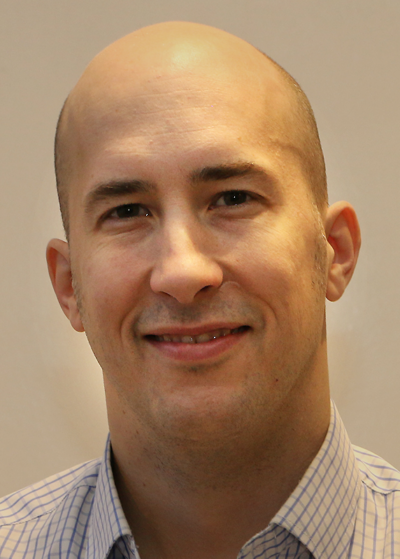
Tobias Sundqvist
- Affiliation: LTH, Department of Computer Science
- Academic supervisors: Volker Krueger, Luigi Nardi
- Research keywords: Computer Vision, Robotics
- Research aim: How to make computer vision pipelines more robust in the context of robotics?
- Publications:
- Email: simon.kristoffersson_lind@cs.lth.se
- Affiliation: Chalmers
- Academic supervisors:
- Research keywords: extreme values
- Research aim: Combination of different anomality detection methods
- Publications: https://scholar.google.com/citations?hl=en&user=ovHxz5IAAAAJ
- Email: hrootzen@chalmers.se
- Affiliation: Linköping University
- Academic supervisors:
- Research keywords: Model-based and data-driven diagnosis and prognosis
- Research aim: Automatic design of model-based, data-driven and hybrid diagnosis systems capable of both detecting and isolating faults. Example of problems studied right now is how to handle non-representative data from faulty cases and how to apply data-driven techniques to modularized systems where all individuals are composed of known modules but in different ways.
- Publications: https://scholar.google.com/citations?hl=en&user=4wcc0cwAAAAJ
- Email: hrootzen@chalmers.se
- Affiliation: Chalmers and Semcon
- Academic supervisors: Christian Berger
- Research keywords: Out-of-distribution detection, vehicle safety, machine learning
- Research aim: The goal of this research is how can we ensure that deep learning models operate correctly in safety critical applications. The ability to reject outlier data when a machine learning model is uncertain is studied by extending the system with outlier detection algorithms that reports how restrictive the outlier detection models must be to fulfill safety-oriented goals.
- Publications: https://scholar.google.com/citations?user=W5sZP1cAAAAJ
- Email:jens.henriksson@semcon.com